2024 European Winter Meeting, Palma de Majorca, Spain: December, 2024
Two-step Parametric Estimation of Binary Treatment Effects in the Presence of Misclassification and Endogeneity
Georgios Marios Chrysanthou
I provide a two-step parametric estimator correcting for misclassification and endogeneity biases in binary treatment effects. Approximate consistency is achieved via modified MLE estimation (MMLE) of the reduced form binary discrete choice model and, modified least squares (MLS) estimation of the structural form which is augmented by a misclassification-corrected control function. The model incorporates unequal/equal misclassification probabilities for false negatives/positives, and estimates misclassification rates without reliance on extraneous information or surrogate measurements. The two-step MLS (2SMLS) estimator outperforms naive instrumental variables estimation (IV) that ignores misclassification, and OLS in terms of bias reduction. If the treatment parameter has the same sign as the error correlation, approximate OLS bias cancellation occurs rendering OLS comparable to 2MSLS if the estimable (error correlation/misclassification) ratio is equal to 1 but this may extend to ratios in [0.5-1] as per simulations. The 2SMLS method provides estimates of the degree of endogeneity and misclassification such that practitioners can assess overall potential bias. Structural identification of the 2SMLS estimator requires a relevant exclusion restriction. The estimator is applied to study the impact of labour market inactivity on social benefit income.
Preview
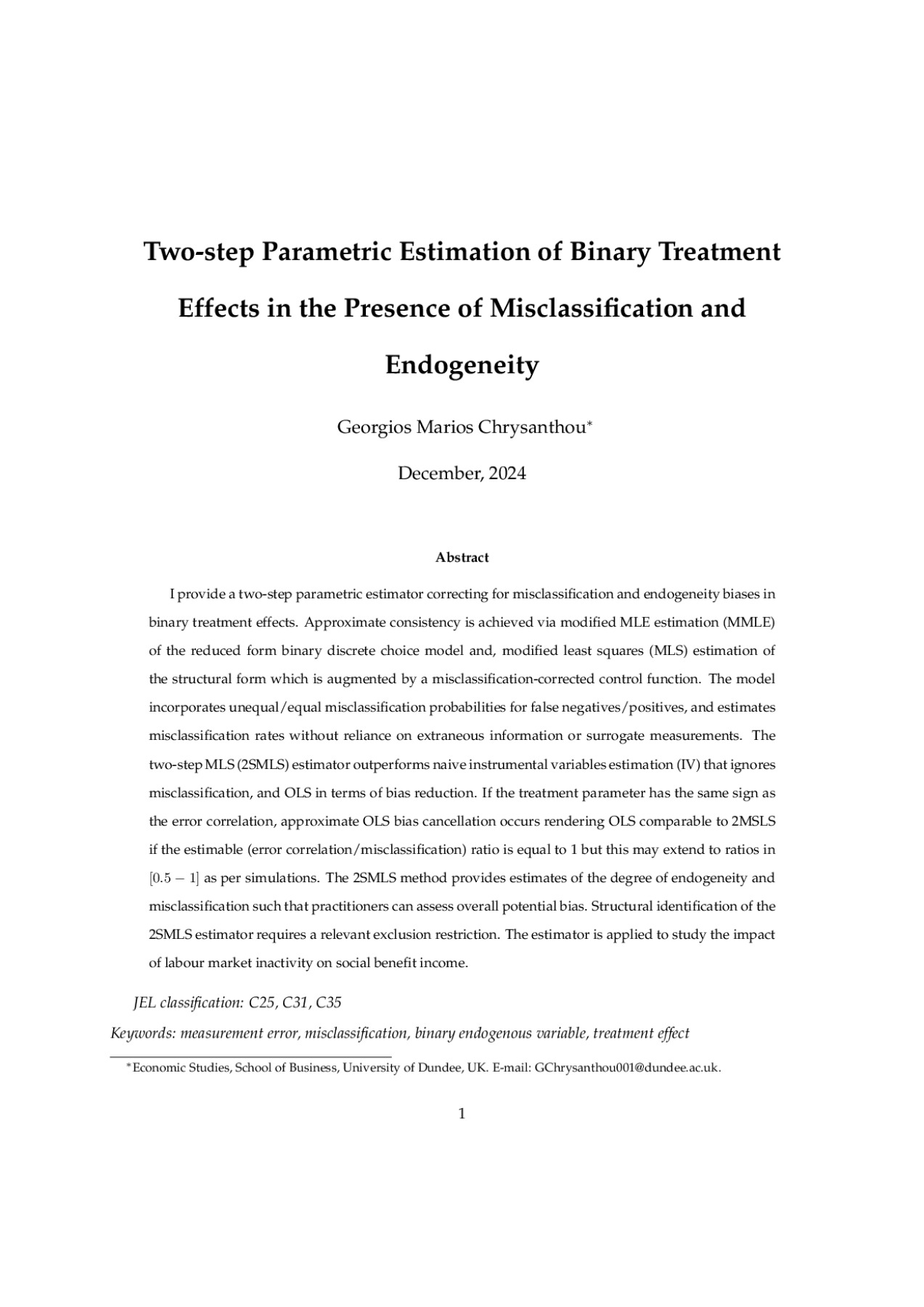




























