2024 North American Summer Meeting: June, 2024
Double Robust Bayesian Inference on Average Treatment Effects
Breunig, Liu, Yu
We propose a double robust Bayesian inference procedure on the average
treatment effect (ATE) under unconfoundedness. Our robust Bayesian approach
involves two important modifications: first, we adjust the prior distributions of
the conditional mean function; second, we correct the posterior distribution of
the resulting ATE. Both adjustments make use of pilot estimators motivated by
the semiparametric in uence function for ATE estimation. We prove asymptotic
equivalence of our Bayesian procedure and efficient frequentist ATE estimators
by establishing a new semiparametric Bernstein-von Mises theorem under double
robustness; i.e., the lack of smoothness of conditional mean functions can be
compensated by high regularity of the propensity score and vice versa. Consequently,
the resulting Bayesian credible sets form confidence intervals with asymptotically
exact coverage probability. In simulations, our double robust Bayesian procedure
leads to significant bias reduction of point estimation over conventional Bayesian
methods and more accurate coverage of confidence intervals compared to existing
frequentist methods. We illustrate our method in an application to the National
Supported Work Demonstration.
treatment effect (ATE) under unconfoundedness. Our robust Bayesian approach
involves two important modifications: first, we adjust the prior distributions of
the conditional mean function; second, we correct the posterior distribution of
the resulting ATE. Both adjustments make use of pilot estimators motivated by
the semiparametric in uence function for ATE estimation. We prove asymptotic
equivalence of our Bayesian procedure and efficient frequentist ATE estimators
by establishing a new semiparametric Bernstein-von Mises theorem under double
robustness; i.e., the lack of smoothness of conditional mean functions can be
compensated by high regularity of the propensity score and vice versa. Consequently,
the resulting Bayesian credible sets form confidence intervals with asymptotically
exact coverage probability. In simulations, our double robust Bayesian procedure
leads to significant bias reduction of point estimation over conventional Bayesian
methods and more accurate coverage of confidence intervals compared to existing
frequentist methods. We illustrate our method in an application to the National
Supported Work Demonstration.
Versions available:
Preview
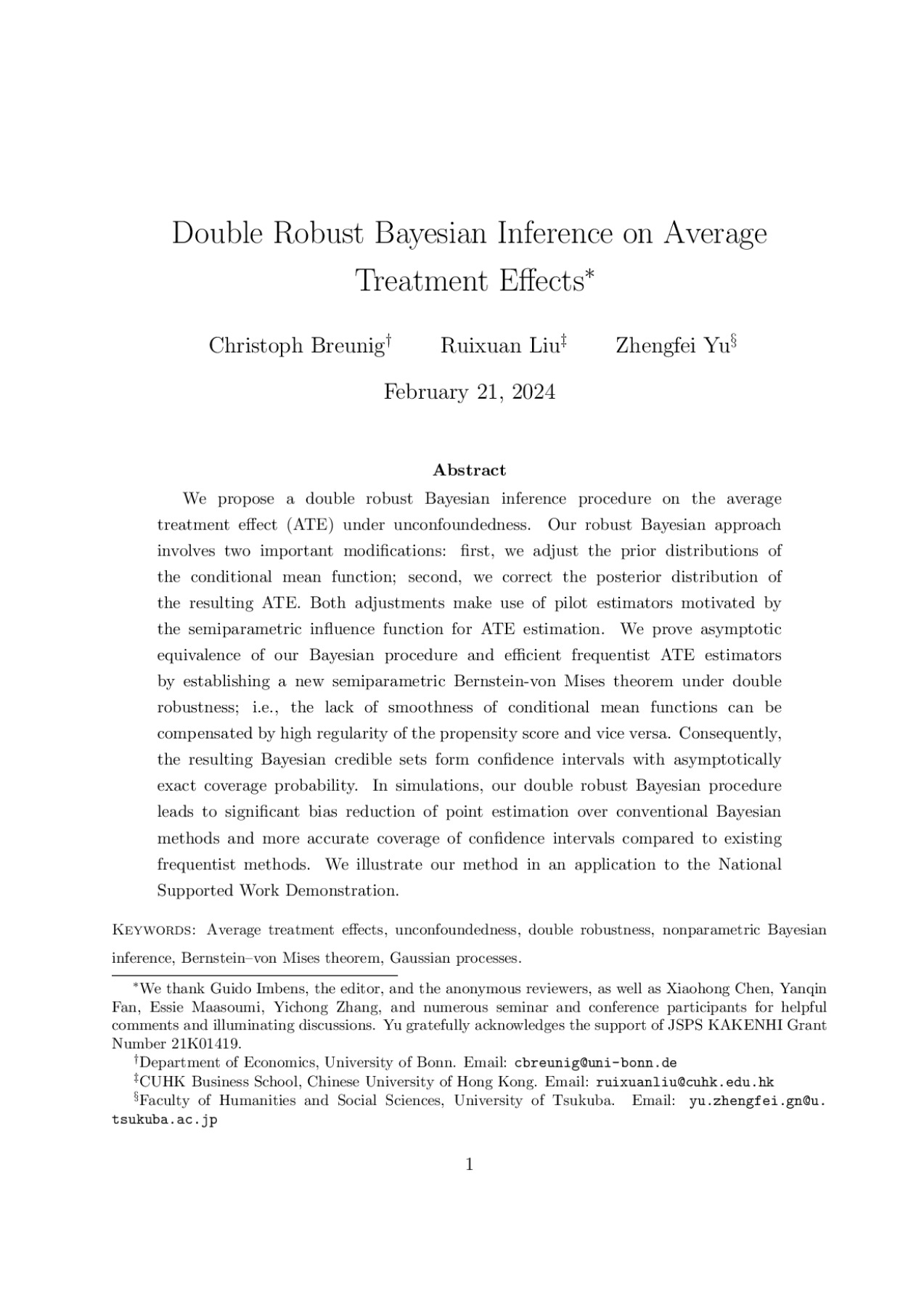



























































